Cycle of Continuous Improvement Higher Education Models
Creating a continuous cycle of improvement with observability
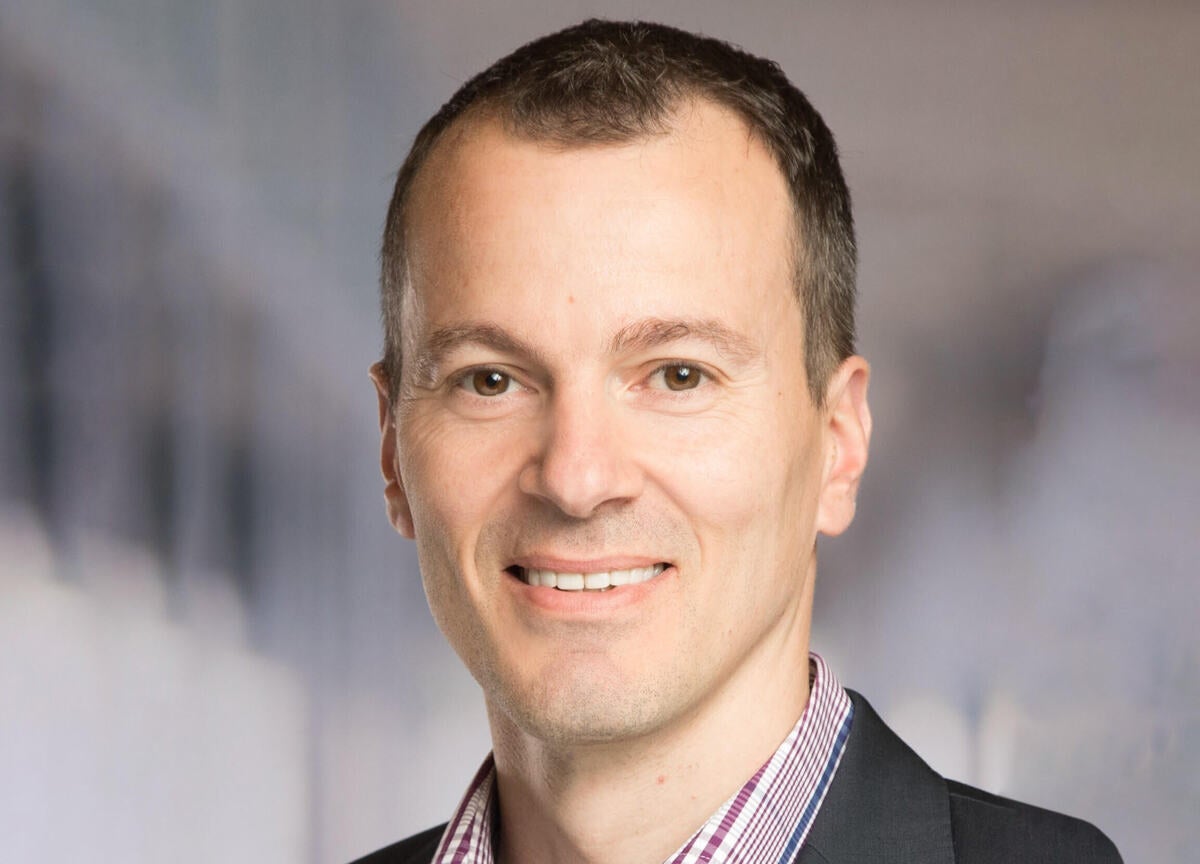
Continuous improvement has been mastered by industry leaders like Jeff Bezos who popularised the term "flywheel" to describe a virtuous cycle of improvement in product delivery and customer experience. Amazon has risen to great heights of success by offering customers competitive prices and swift delivery, while taking feedback on board to improve the future of its business.
This approach creates and maintains a continuous cycle of improvement. Using customer feedback, Amazon is able to improve the future of its business and drive more traffic to its platform. This enables the growth of more third-party sellers, and in turn allows for a wider selection of goods to be made available, attracting more customers and increasing customer lifetime value.
So what does this have to do with observability? Software engineering teams use observability to understand complex digital systems, including the 'what,' 'when' and 'why' of problems. Through looking at system outputs, such as metrics, events, logs, and traces, observability creates a clear picture of how a system is behaving.
What's unique about observability is that it can facilitate its own flywheel. Unlike reporting systems that roll up insights at the end of a weekly or monthly reporting period, observability provides a real-time continuous feedback loop that enables problems to be anticipated and resolved much sooner.
Detect subtle changes and do something about them
In the past, technology like observability was often justified because of a compelling event, such as when an entire network, application or server farm crashed. With cloud computing and resilient cloud-native software architectures, these issues are less of a concern today.
In the cloud-native era, customer journeys often depend on many more microservices and systems, which means the collective performance and availability of the services determines the performance and availability of customer experiences. To counteract this risk, observability practitioners should spend more time detecting and investigating subtle changes in performance and availability to avoid the magnifying effect on customer experience. Let's demonstrate with a simple example.
Say that a customer journey depends on 10 microservices with a particular performance Service Level Objective (SLO) of 99.9% per service per month. If these service-level targets are suppressed at different points in time and all are required to service customers, the worst-case performance becomes 99.9% to the power of 10. This represents an additional 395 minutes of suppressed performance versus 43 minutes if all services were performing at 99.9%. The moral of the story is this: we need to pay attention to subtle changes in service performance and availability because each percentage point lost can have an exponential impact on customer experience.
Always measure from the customer's point of view
To truly quantify customer experience we must measure the customer journey's from their point of view. This will create an understanding of how the software and architecture behaves when exposed to vastly different client conditions (e.g. devices and connectivity options). For example, an application running on a 3 year old iPhone using 4G will perform differently to a new generation iPhone using 5G connectivity. It is not possible to test all permutations. Observing the production customer experience allows the real-world customer experience to be quantified so that data-driven decisions can be made. New Relic customer Ansarada is an example of this.
The Australian developer of AI-powered virtual data rooms deployed New Relic's observability platform to maintain the robust nature of its website. Companies such as BHP, VMWare, Virgin, BPAY, Goldman Sachs, UBS, and Credit Suisse rely on Ansarada to ensure their sensitive deals and information are handled in an environment of security and reliability. High-pressure environments like these mean that seconds of lag, minor delays, or downtime are detrimental.
New Relic supported the business to reduce the time to detect and fix issues to an average of just 30 minutes—a 90% improvement on mean time to recovery (MTTR). This ability to rapidly diagnose issues and facilitate recovery has a tremendous impact on customers, and allows teams to invest their time on innovating instead of firefighting.
Facilitating innovation
In a cloud-native world, innovation and iterating quickly on software needs to be balanced with the needs of established customers, who demand stable and reliable services. Separating releases from deployments using a single trunk approach to development, and leveraging concepts such as feature flags and progressive rollouts, allows DevOps teams to speed up development and deployment velocity while limiting the number of customers impacted by a change or new feature that has the potential to introduce problems.
To facilitate this approach, the distributed traces and metrics provided by observability platforms like New Relic can be decorated with custom metadata. This allows engineers to use the observability platform to track and quantify the impact of changes and behaviour in real-time across cohorts; in turn ensuring that high performance teams can consistently achieve peak performance and stability by observing and reacting to unplanned scenarios as quickly as possible.
By using observability as a way to ensure consistent service delivery, tech teams are able to create their own flywheel of continuous improvement which considers the customer experience and avoids even the smallest delays. Through creating a real-time continuous feedback loop forged by observability, technology leaders have the opportunity to look at the subtle changes impacting their operating environment and take steps to remedy these before their customers notice. This creates a culture of optimisation and innovation and sets tech teams apart from their counterparts.
Authored by Peter Marelas, New Relic APJ Chief Architect
Copyright © 2021 IDG Communications, Inc.
franklinpural1985.blogspot.com
Source: https://www.computerworld.com/article/3644890/creating-a-continuous-cycle-of-improvement-with-observability.html
0 Response to "Cycle of Continuous Improvement Higher Education Models"
Post a Comment